- Home
- Assessments
- Bioregional Assessment Program
- Methods
- Propagating uncertainty through models
- 3 Requirements and assumptions
This chapter summarises the main requirements for a viable and robust uncertainty analysis methodology for bioregional assessments (BAs), and the high-level decisions and assumptions that underpin this methodology.
3.1 Requirements
3.1.1 Transparent and reproducible
Underlying the BA is the requirement that every outcome needs to be reproducible and each step or process that leads to an outcome needs to be documented in a transparent manner so as to allow public scrutiny. These terms are defined in BAs as follows:
- transparency: a key requirement for the Bioregional Assessment Programme, achieved by providing the methods and unencumbered models, data and software to the public so that experts outside of the Assessment team can understand how a bioregional assessment was undertaken and update it using different models, data or software
- reproducibility: the extent to which materially consistent results are obtained when experts outside of the Assessment teams redo part or all of a bioregional assessment using the same methods, models, data and software, but different computer systems.
These requirements of transparency and reproducibility require researchers in the BA to be diligent in recording in detail the reasoning, the uncertainty associated with any information or model used in BA, and the chain of models that led to a prediction and the source of all the data and information that fed into that chain of models.
Section 3.1 provides guidance on how to characterise and report data and model uncertainty that will feed into the modelling chain. After establishing these uncertainties, it is key that the methodology outlined in this document is adhered to for the propagation of the uncertainty to the prediction of interest (that is the impact on the asset and/or landscape class or the hydrological response variable).
3.1.2 Generic
Due to the nature of the research question, the research in BA inevitably will include various scientific disciplines and work across very different scales. Each scientific discipline has different traditions in handling uncertainty, inspired by the boundary conditions imposed on the statistical techniques applicable to the discipline, by the typical availability of data and by the system linearity and dimensionality.
In order to use the same methodology throughout the BA process, the uncertainty analysis methodology needs to be able to accommodate and integrate various disciplines and different spatial and temporal scales. The methodology therefore will need to be flexible and generic and thus model-independent.
3.1.3 Probabilistic
There are multiple ways of analysing risks associated with future activities. The companion submethodology M11 (as listed in Table 1) for hazard analysis (Ford et al., 2016) opts to apply a risk analysis based upon probabilistic estimates of the uncertainties associated with the predicted impacts of coal mining and coal seam gas development on water and water-dependent assets. This obviously implies that the uncertainty analysis needs to provide probabilistic estimates of the uncertainty of the impact on assets and/or landscape classes or hydrological response variables.
3.1.4 Data and model availability
The quantity and quality of data available to inform the BA is, to say the least, variable. It will not only vary between bioregions, in which for instance the Northern Inland Catchments bioregion has more and more-reliable data than the Lake Eyre Basin bioregion, it will also vary within a bioregion. For example, there might be a high density of observations for shallow groundwater levels, but hardly any data for groundwater pressure in deep aquifers.
The methodology will need to be able to produce an estimate of uncertainty, even if there is no or limited data available. The methodology will also need to accommodate the situation where hard data are only available for parts of the system. While it is essential that all available data are used to constrain the models, care has to be taken not to give overly great weight to small datasets or unrepresentative data in order to avoid bias in the predictions.
Related to the data availability issue is the model availability. The BA project will not be able to create comprehensive, complex models for each aspect of each bioregion. In some regions existing models, created by or for state agencies or mining companies, will be available. As the intellectual property of these models does not reside with the BA partners, it is likely that conditions will be imposed on the BA team as to what extent the model and model results can be used. An example would be that BA is allowed to use the model results, but cannot run the model with changed parameter values or boundary conditions. The methodology will need to accommodate such limitations on the use of models and results.
3.1.5 Practical
The four requirements discussed above condition the science needed to assess uncertainties in the predictions. The reality of time and resources available for the BA, however, require that practical considerations be taken into account as well.
Each researcher in the BA is expected to have a working knowledge of the uncertainty methodology. It is, however, unrealistic to expect every researcher to be across all the mathematical and statistical detail of the methodology, let alone have the software engineering capabilities to apply the methodology to their domain. The methodology therefore needs to be designed so that it can be used, with limited training, by all researchers within the BA.
Additionally, while considerable computing infrastructure is available for the project, resources are not unlimited. The implementation of the uncertainty methodology therefore needs to ensure it is computationally feasible within the time frames agreed in the project plans for individual bioregions.
3.2 Assumptions
3.2.1 Every aspect of the model chain needs scrutiny
The default position when starting to address the question of how coal seam gas extraction and coal mining development will affect an asset is to consider each aspect of the conceptual model and the resulting chain of models as uncertain.
Many of these aspects will be able to be expressed in probabilistic terms. The uncertainty of continuous variables, such as hydraulic conductivity, river discharge or fish biomass, can be directly expressed as a probability density function. In the case of categorical variables, such as fault presence or vegetation type, uncertainty can be expressed as a probability of occurrence. Any aspect that can be expressed in such probabilistic terms will be incorporated formally in the uncertainty analysis process (Section 4.2 and Section 4.3).
However, discrete choices and simplifications are unavoidable in developing conceptual and physical models and it will not always be possible to assign probabilities to competing choices. Section 4.1.1 will discuss in more detail how to assess and document these discrete choices and model assumptions.
3.2.2 Problem can be compartmentalised
The conceptual model will provide causal pathways between coal resource development and impacts on assets. In theory, and as advocated by Voinov and Shugart (2013), it is possible to translate that conceptual model into a chain of physical models and, with the help of a workflow system, carry out an uncertainty analysis of the integrated system.
This will place enormous strain on the logistics of the BA process as it will require all the models that are part of the model chain to be available before the uncertainty analysis can start. Enormous gains in efficiency and tractability can be achieved if the uncertainty analysis can be compartmentalised (i.e. if the model chain can be split up into sub-processes for which the uncertainty analysis can be carried out sequentially but separately).
The main criterion to subdivide the chain of models is the absence of feedback loops. Groundwater models will provide an estimate of baseflow to river models, which in turn affects the river stage, which often is a boundary condition for groundwater models. Such an intimate link between models implies that they cannot be separated, as a change in one model has the potential to affect the other model. A change in hydrology has the potential to change the ecology of that catchment. It is however unlikely that this change in ecology will change the hydrology sufficiently to invalidate the earlier hydrological change simulation. This implies that hydrological models can be isolated from the ecological models where outputs of the former (and associated uncertainty estimates) become inputs for the latter. Note that any external major changes in ecology due to coal resource development, such as land clearance for open-cut mining of coal, are part of the change in stress to the hydrologic models, for instance by specifying a change in runoff in a catchment.
Again, it is paramount to record and document the reasoning and justification for compartmentalising a conceptual model. If there are good reasons to expect important feedback loops between the ecology and the hydrology these should be identified as part of the conceptual modelling. If it is possible to include this feedback in the model chain then that should happen. If not, then it should be, at the very least, acknowledged as something to watch and a potential gap.
3.2.3 Well-defined hydrological response variables
It is important to establish well-defined hydrological response variables, explicitly defined in space and time, for each sub-model, that can: (i) be used to summarise the results of the numerical modelling and (ii) support reasoned explanations for the potential changes in assets or landscape classes (refer to companion submethodology M03 (as listed in Table 1) for assigning receptors to water-dependent assets (O’Grady et al., 2016)) and (iii) be used in the receptor impact modelling (refer to companion submethodology M08 (as listed in Table 1) about receptor impact modelling).
The physical models, hydrogeological and hydrological, will produce both time series and/or maps of change in groundwater level, flux or river flow. From a practical perspective, due to the high dimensionality of the model output, it is not possible to calculate uncertainty estimates for each grid cell and each time step of the model. A limited number of hydrological response variables therefore need to be defined that either summarise the spatial and temporal output of the model or are representative for the spatial and temporal output of the model. An example of this is using the following hydrological response variables to summarise the time series of drawdown at a model node:
- dmax: maximum difference in drawdown for one realisation within an ensemble of groundwater modelling runs, obtained by choosing the maximum of the time series of differences between two futures
- tmax: year of maximum change.
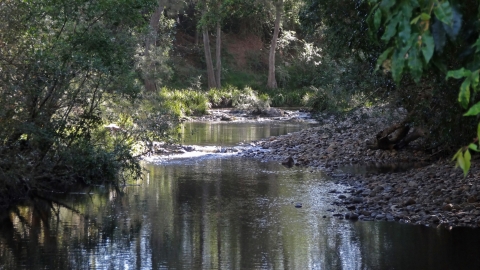